This is a pleasant (but a war) story on how UX was perceived when I joined the client's product team, what the team’s goal is, and what has changed in terms of doing research after a little less than a year - focusing on a particular qualitative research method (customer interviews), and here's how we used tagging taxonomy as a form of structuring data.
The team that I’m currently a part of is called the Customer Support team, and our mission is to reduce costs and increase efficiency by reducing unwanted inbound volume to the customer care centre and reducing the number of returns to the store.
Unwanted inbound volume (in our case) means that we want to minimise the number of calls from customers asking for information that can be found on the website, their receipt, order confirmation, or delivery tracking/repair tracking.
How was our team working on reducing unwanted inbound volume to customer service? By developing self-services that customers can use to get answers about either the delivery status or their repair ticket, and also by digitalising some actions, e.g., rescheduling the delivery date.
The client had plenty of quantitative data collected across a few platforms such as Hotjar, Happy or Not, Google Analytics , but not much had been done in terms of analysis.
After scanning piles of customer feedback for all four countries and checking the user flow of contact pages, we focused on B2X ratings and reviews - informing team members monthly about the ratings of individual support pages, detecting possible technical issues, and revealing possible new customer pain points.

pages of Elkjøp.
From the perspective of a UX analyst trying to dig deeper into the problem of customers calling customer service with relatively easy questions or issues that are supposed to be answered on the support pages of an ecommerce site, first of all we needed to investigate current architecture on support pages and the data that had been documented.
We continued with co-listenings where we observed the communication between the call centre agent and the customer, focusing on the contact reasons for why they called and the context behind it. The most frequent contact reasons were one of the starting points for our interviews. Even though we have a lot of quantitative data, we didn’t have any insight into the customer’s behaviour and their past experiences - and feelings.
And we did it! We conducted user interviews with semi-opened questions. Rather than focusing solely on numbers, we wanted to get insight into past experiences that the customer might have had with the website, to investigate what happened and to give them a chance to give us relevant feedback. We spoke with 21 people, and 18 of them had experience when contacting customer service. Every such experience was (at least) at first, negative.
But the job was not done at that point - conversations needed to be listened to again, transcriptions needed to be made, and data to be sorted. For this, I used a basic plan from Dovetail, which allows you to analyse, store, and share your customer research in a collaborative platform. So here was the tricky part. I came into an organisation that gave a lot of importance to numbers, percentages. Percentages that could be easily shown with a graph and connected to a business case - and there’s nothing wrong with that. On the other hand, there was little to no experience on the subject of talking to customers. So it was crucial at this step to create a tagging taxonomy.
A taxonomy is a system one uses to classify and organise raw research data.
It helps you:
• Find research data and insights quickly under one tag
• Discover new insights
• Connect multiple researches (done with the same method) into a valuable repository over time.
Taxonomies are like an organism. They can change and evolve over time. For easier work, make sure that renaming, merging, or splitting them up is somewhat easy. Use global tags that are relevant beyond a single study.
Flat taxonomy is simply a list of items. It has only high-level categories.
Pros: Easy to build the initial taxonomy, tags can be applied to feedback more consistently and accurately as there are fewer tags to choose from, and great in situations where the data volume is relatively low.
Cons: Difficult to compare tags as they can differ in their level of abstraction, not sustainable when using a large number of tags, difficult to expand without overlapping.
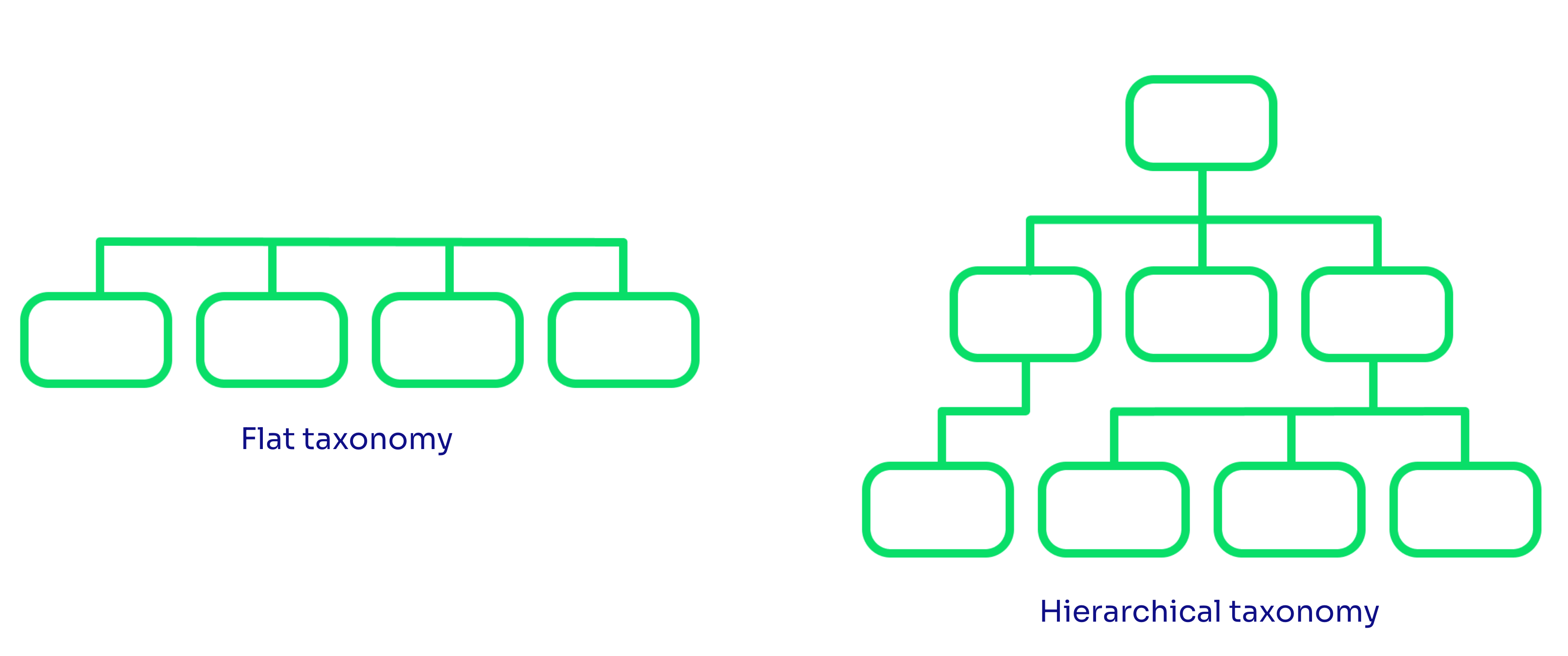
Hierarchical taxonomy allows a hierarchical arrangement of tags. Individual tags within the hierarchy are arranged in order of abstraction. Moving up the hierarchy means expanding the tag or concept, while moving down the hierarchy means refining the tag or concept.
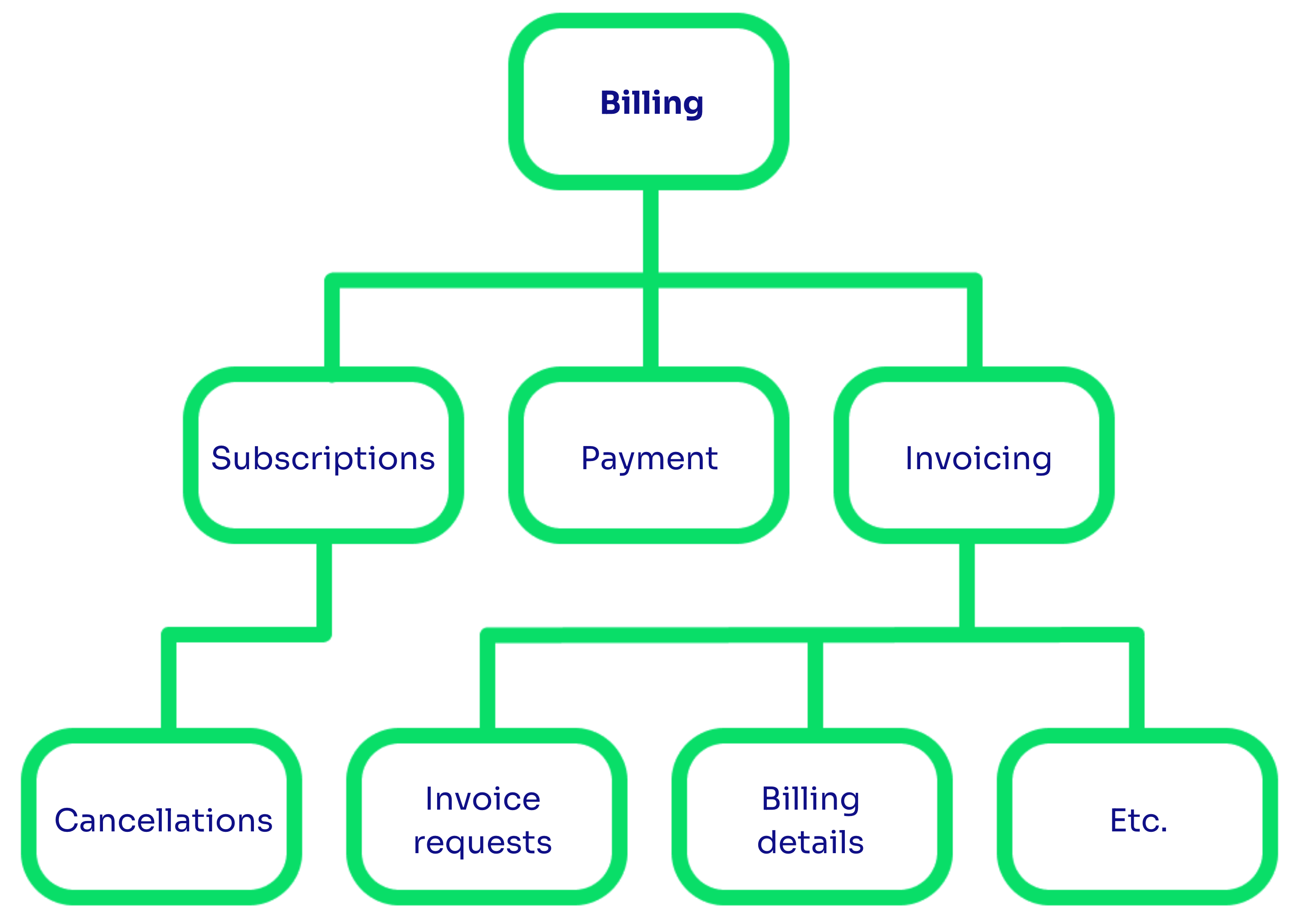

After going through all transcriptions, an initial tagging taxonomy was created. But it was too specific and not expandable. So for easier future tagging, I created a repository below.

This kind of repository was much more scannable and visible.
• Plan for a learning curve. I t can get frustrating to learn a tool in the same time that you want to complete the task. Baby steps are important.
• Don't create new tags for every project. instead, think of next steps ahead - are the tags that you will create now, be expandable?